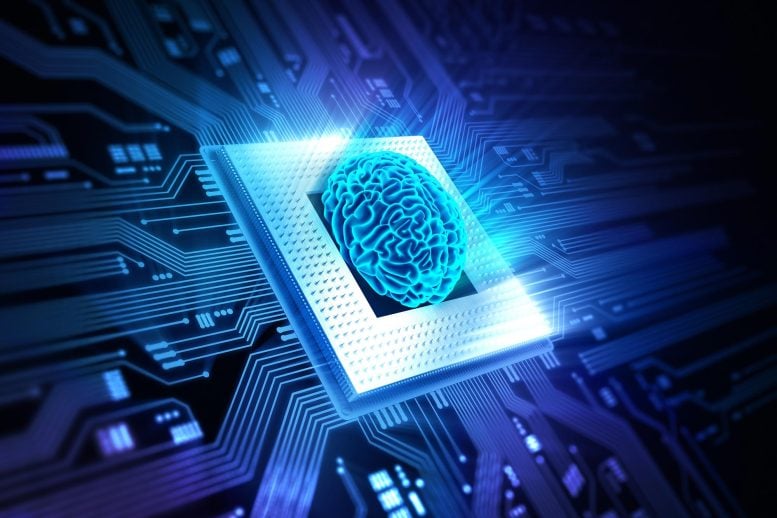
NUS researchers have shown that a single trans istor can replicate both neural and synaptic behaviors, marking a significant step toward brain-inspired computing.
Researchers at the National University of Singapore (NUS) have shown that a single, standard silicon transistor, the core component of microchips found in computers, smartphones, and nearly all modern electronics, can mimic the functions of both a biological neuron and <span class="glossaryLink" aria-describedby="tt" data-cmtooltip="
” data-gt-translate-attributes=”[{"attribute":"data-cmtooltip", "format":"html"}]” tabindex=”0″ role=”link”>synapse when operated in a nontraditional manner.
The study, led by Associate Professor Mario Lanza from the Department of Materials Science and Engineering at NUS’s College of Design and Engineering, offers a promising path toward scalable, energy-efficient hardware for artificial neural networks (ANNs). This advancement marks a significant step forward in neuromorphic computing, a field that aims to replicate the brain’s efficiency in processing information. The research was published in Nature on March 26, 2025.
Putting the brains in silicon
The world’s most sophisticated computers already exist inside our heads. Studies show that the human brain is, by and large, more energy-efficient than electronic processors, thanks to almost 90 billion neurons that form some 100 trillion connections with each other, and synapses that tune their strength over time — a process known as synaptic plasticity, which underpins learning and memory.
For decades, scientists have sought to replicate this efficiency using artificial neural networks (ANNs). ANNs have recently driven remarkable advances in <span class="glossaryLink" aria-describedby="tt" data-cmtooltip="
” data-gt-translate-attributes=”[{"attribute":"data-cmtooltip", "format":"html"}]” tabindex=”0″ role=”link”>artificial intelligence (AI), loosely inspired by how the brain processes information. But while they borrow biological terminology, the similarities run only skin deep — software-based ANNs, such as those powering large language models like ChatGPT, have a voracious appetite for computational resources, and hence, electricity. This makes them impractical for many applications.
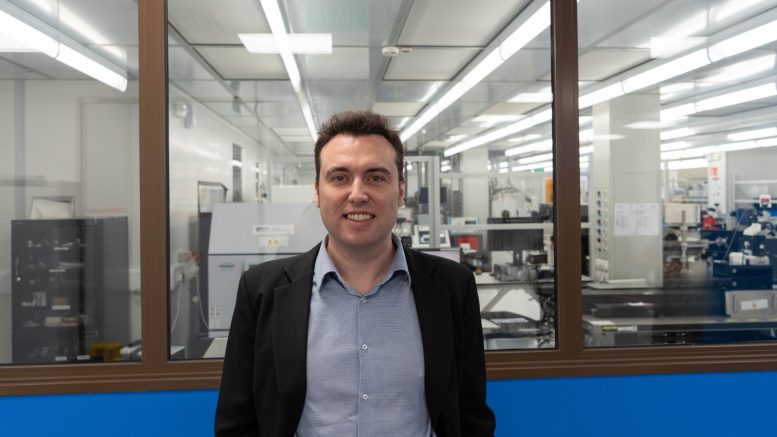
Neuromorphic computing aims to mimic the computing power and energy efficiency of the brain. This requires not only re-designing system architecture to carry out memory and computation at the same place — the so-called in-memory computing (IMC) — but also to develop electronic devices that exploit physical and electronic phenomena capable of replicating more faithfully how neurons and synapses work. However, current neuromorphic computing systems are stymied by the need for complicated multi-transistor circuits or emerging materials that are yet to be validated for large-scale manufacturing.
“To enable true neuromorphic computing, where microchips behave like biological neurons and synapses, we need hardware that is both scalable and energy-efficient,” said Professor Lanza.
A Breakthrough Using Standard Silicon
The NUS research team has now demonstrated that a single, standard silicon transistor, when arranged and operated in a specific way, can replicate both neural firing and synaptic weight changes — the fundamental mechanisms of biological neurons and synapses. This was achieved through adjusting the resistance of the bulk terminal to specific values, which allow controlling two physical phenomena taking place into the transistor: punch through impact ionization and charge trapping. Moreover, the team built a two-transistor cell capable of operating either in neuron or synaptic regime, which the researchers have called “Neuro-Synaptic Random Access Memory”, or NS-RAM.
“Other approaches require complex transistor arrays or novel materials with uncertain manufacturability, but our method makes use of commercial CMOS (complementary metal-oxide-semiconductor) technology, the same platform found in modern computer processors and memory microchips,” explained Professor Lanza. “This means it’s scalable, reliable, and compatible with existing semiconductor fabrication processes.”
Through experiments, the NS-RAM cell demonstrated low power consumption, maintained stable performance over many cycles of operation, and exhibited consistent, predictable behavior across different devices — all of which are desired attributes for building reliable ANN hardware suited for real-world applications. The team’s breakthrough marks a step change in the development of compact, power-efficient AI processors that could enable faster, more responsive computing.
Reference: “Synaptic and neural behaviours in a standard silicon transistor” by Sebastian Pazos, Kaichen Zhu, Marco A. Villena, Osamah Alharbi, Wenwen Zheng, Yaqing Shen, Yue Yuan, Yue Ping and Mario Lanza, 26 March 2025, Nature.
DOI: 10.1038/s41586-025-08742-4